Glossary of Industrial Maintenance Technology
It can be challenging to understand what all the terms for industrial maintenance technologies mean. IIoT, Industry 4.0, AI, PdM 4.0. We try to distill it down to a simple glossary.
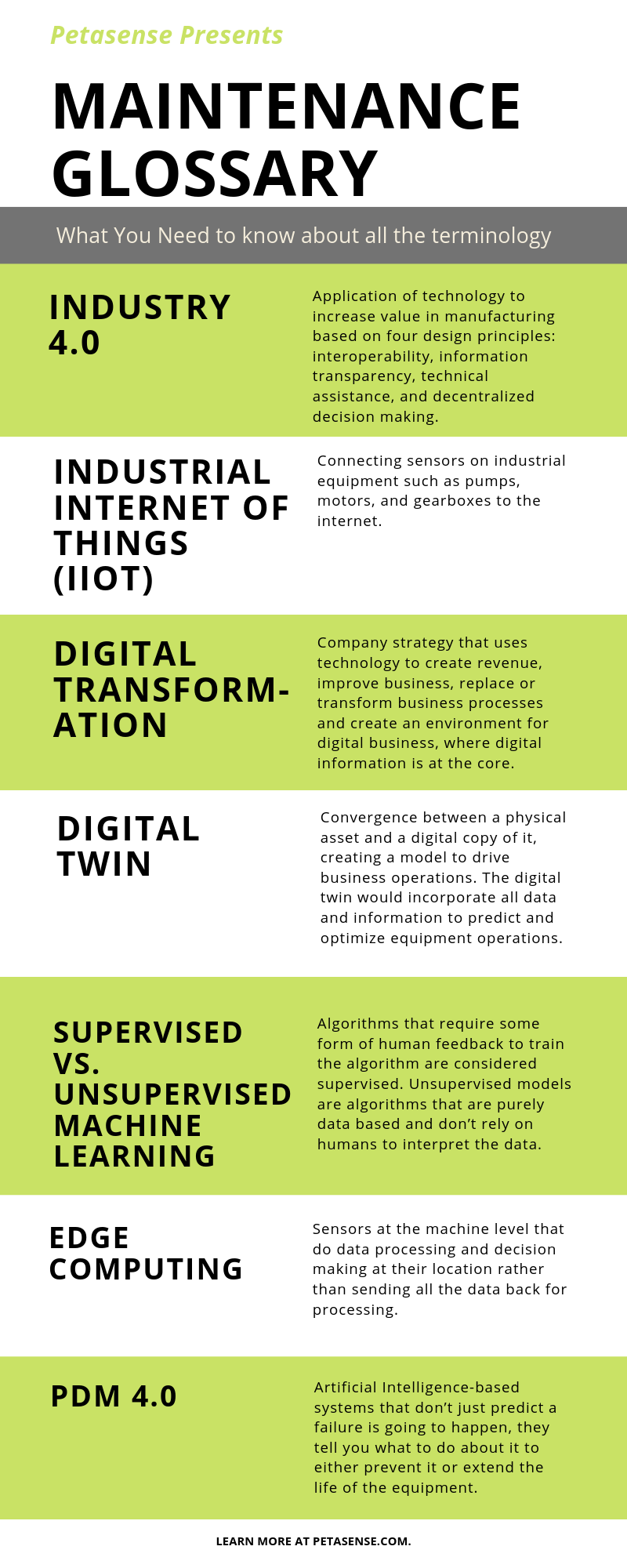
Industry 4.0
A term coined by the German government as part of it’s High Tech strategy 2025 that centers around how technology can be used to increase value in manufacturing (from supply chain to automation). It operates across four design principles: interoperability, information transparency, technical assistance, and decentralized decision making. Many people have criticized it as being too theoretical and academic.
Industrial Internet of Things (IIoT)
General Electric is credited with coining the term, which has been driven largely by companies rather than government, and focuses on connecting equipment to the internet. People have become familiar with the Internet of Things over the last few years, which is about connecting smart (e.g. refrigerator) devices to the internet. IIoT connects sensors on industrial equipment such as pumps, motors, and gearboxes to the internet. This opens a large opportunity for improving efficiency, safety, and productivity.
Many people confuse IIoT and Industry 4.0. The key difference is the scope. Industry 4.0 looks at technology automating the entire chain (e.g. how the customer orders, manufacturing, supply chain, delivery), while IIoT is about connecting the physical production machines to the internet.
Digital transformation
A company-wide strategy centering around how to use technology to create revenue, improve business, replace or transform business processes and create an environment for digital business, where digital information is at the core. It applies to all elements of the business, not only production/manufacturing.
Digital twin
It centers on the convergence between a physical asset and a digital copy of it, creating a model to drive business operations. The digital twin incorporates all data and information to predict and optimize equipment operations. For example, it could take asset data from a multitude of systems, such as the CMMS, condition monitoring, SCADA, OEM specification, and much more. Few companies have implemented the digital twin, and the resources needed to implement it make it prohibitive. Imagine, if you will, needing to have all the original drawings, as well as any modifications that were made to the equipment, a complete and accurate CMMS, as well as all the human-contained knowledge.
Prescriptive Maintenance
Another term that is becoming popular within the maintenance realm is Prescriptive Maintenance, which refers to Artificial Intelligence-based systems that don’t just predict a failure is going to happen, they tell you what to do about it to either prevent it or extend the life of the equipment.
PdM 4.0
Similar to Prescriptive Maintenance, this refers to the evolution of Predictive Maintenance, or “applying the power of machine learning techniques to identify meaningful patterns in vast amounts of data and generate new, actionable insights for improving asset availability.”
Supervised models
Within the field of Artificial Intelligence, there is a whole range of terminologies as well. One of the most common is the distinction between Supervised and Unsupervised models. Algorithms that require some form of human feedback to train the algorithm are considered supervised. In the case of Predictive Maintenance, this could be the knowledge that is fed into the algorithm connecting the failure pattern with the underlying failure mode. In other words, the algorithm can tell there is a problem, but it doesn’t know that it is caused by misalignment.
Unsupervised models
Algorithms that are purely data based and don’t rely on humans to interpret the data. For example, anomaly detection or recognizing that multiple parameters are out of their normal range can be done entirely by an algorithm and much faster and better than a human could do. That is what it does best!
Edge computing or Edge devices
This refers to sensors at the machine level that do data processing and decision making at their location rather than sending all the data back for processing. It is especially useful in applications where speed of decision making is important, lower computing power is required, and / or where there are bandwidth or connectivity issues.